Unlocking Earth's Secrets: A Comprehensive Guide to Land Cover Classification of Remotely Sensed Images

Land cover classification is a fundamental aspect of remote sensing, enabling us to decipher the intricate tapestry of Earth's landscapes. By analyzing remotely sensed images, we unlock a wealth of information about the distribution and characteristics of مختلف land cover types, expanding our understanding of our planet and its ecosystems.
4.2 out of 5
Language | : | English |
File size | : | 11194 KB |
Text-to-Speech | : | Enabled |
Screen Reader | : | Supported |
Enhanced typesetting | : | Enabled |
Print length | : | 281 pages |
This comprehensive guide will immerse you in the world of land cover classification, providing a thorough exploration of its techniques, applications, and the latest advancements. Whether you're a seasoned professional or a curious novice, this article will equip you with the knowledge and insights to harness the power of remotely sensed images for land cover analysis.
Understanding Land Cover
Land cover refers to the physical and biological characteristics of Earth's surface, including vegetation, water bodies, urban areas, and agricultural lands. It encompasses the dominant materials, physical structures, and biological communities present in a specific area.
Land cover is crucial for understanding Earth's ecosystems, climate patterns, and human activities. Changes in land cover, such as deforestation or urbanization, can have far-reaching impacts on biodiversity, carbon cycling, and water resources.
Remote Sensing: A Window to Earth's Landscapes
Remote sensing allows us to observe Earth's surface from a distance, using sensors mounted on satellites or aircraft. These sensors collect data in various wavelengths, including visible light, infrared, and microwaves, providing us with a comprehensive picture of land cover characteristics.
Remotely sensed images offer a unique perspective, capturing vast areas in a single frame and enabling the monitoring of land cover changes over time. They provide a valuable tool for land cover classification and a wealth of information for environmental management and decision-making.
Techniques for Land Cover Classification
Land cover classification involves identifying and categorizing different land cover types within remotely sensed images. Various techniques are employed to achieve this, ranging from traditional supervised classification to advanced machine learning algorithms.
Supervised Classification
Supervised classification is a pixel-based approach where each pixel in the image is assigned to a specific land cover class. Training data, consisting of known land cover types, is used to train a classifier that can then be applied to the entire image.
Unsupervised Classification
Unsupervised classification, on the other hand, does not require training data. Instead, algorithms are used to group pixels based on their spectral similarity, resulting in clusters that represent different land cover types.
Hybrid Classification
Hybrid classification methods combine supervised and unsupervised approaches to leverage the strengths of both techniques. They involve an initial unsupervised classification, followed by a supervised classification to refine the results.
Machine Learning and Deep Learning
Recent advancements in machine learning and deep learning have revolutionized land cover classification. These algorithms can automatically extract features from remotely sensed images, making them more efficient and accurate than traditional methods.
Machine learning algorithms, such as support vector machines and decision trees, have shown promising results in land cover classification. Deep learning algorithms, particularly convolutional neural networks (CNNs),have achieved state-of-the-art performance, outperforming traditional methods in complex and large-scale classification tasks.
Applications of Land Cover Classification
Land cover classification has a wide range of applications in various fields, including:
Natural Resource Management
Land cover classification supports the management of forests, wetlands, and other natural resources. It aids in identifying and monitoring critical habitats, assessing biodiversity, and implementing conservation measures.
Environmental Monitoring
By tracking changes in land cover over time, land cover classification enables the detection of deforestation, urbanization, and other environmental impacts. This information is vital for environmental impact assessments, climate change monitoring, and disaster response.
GIS and Spatial Analysis
Land cover classification data is often integrated with geographic information systems (GIS) for spatial analysis. It allows for the exploration of relationships between land cover and other geographic factors, such as topography, soils, and infrastructure.
Land Use Planning
Land cover classification is essential for land use planning and zoning. It provides insights into the current and potential land uses, helping to make informed decisions about development, conservation, and infrastructure.
Land cover classification of remotely sensed images is a powerful tool that empowers us to gain a comprehensive understanding of Earth's landscapes. By embracing the latest techniques and advancements, we can unlock valuable insights into land cover dynamics, monitor environmental changes, and make informed decisions for sustainable land management and conservation.
This guide has provided an in-depth exploration of land cover classification, its techniques, applications, and the transformative role of machine learning and deep learning. As the field continues to evolve, we can expect even more innovative and powerful approaches for classifying land cover and unraveling the secrets of our planet.
4.2 out of 5
Language | : | English |
File size | : | 11194 KB |
Text-to-Speech | : | Enabled |
Screen Reader | : | Supported |
Enhanced typesetting | : | Enabled |
Print length | : | 281 pages |
Do you want to contribute by writing guest posts on this blog?
Please contact us and send us a resume of previous articles that you have written.
Book
Novel
Page
Chapter
Text
Story
Genre
Reader
Library
Paperback
E-book
Magazine
Newspaper
Paragraph
Sentence
Bookmark
Shelf
Glossary
Bibliography
Foreword
Preface
Synopsis
Annotation
Footnote
Manuscript
Scroll
Codex
Tome
Bestseller
Classics
Library card
Narrative
Biography
Autobiography
Memoir
Reference
Encyclopedia
William A Kappele
Tom Dreesen
Peter Loel Boonshaft
Pravin Banker
Tony Bennis
Torrey Seland
Karen Schless Pressley
Peter Loshin
Postremo Vate
Wendy Rowe
Pierre Teilhard De Chardin
Peg Dawson
Nj Mackay
Patricia R Liehr
Philip Alexander
Wendy Leeds Hurwitz
Peter G Tsouras
Polly Williamson
Stephen Gose
Paola Berenstein Jacques
Light bulbAdvertise smarter! Our strategic ad space ensures maximum exposure. Reserve your spot today!
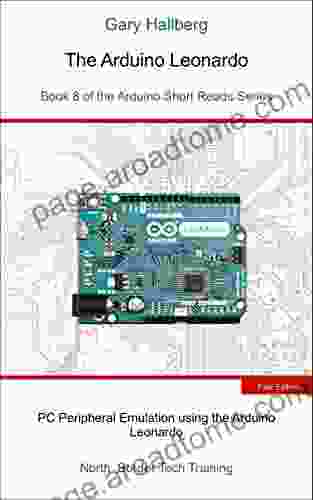

- Abe MitchellFollow ·3.6k
- Javier BellFollow ·13k
- Earl WilliamsFollow ·14.8k
- Theodore MitchellFollow ·14.2k
- Isaiah PowellFollow ·3k
- Benjamin StoneFollow ·16.9k
- H.G. WellsFollow ·8.9k
- Roy BellFollow ·16.4k
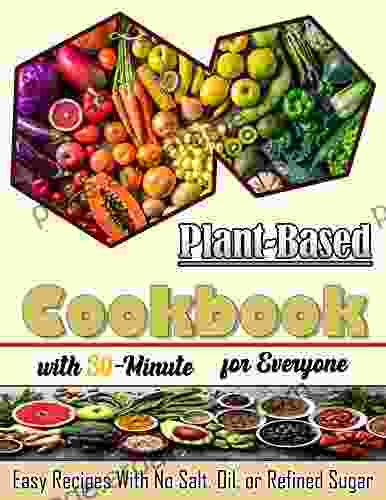

Nourishing Delights: Easy Recipes Without Salt, Oil, or...
Are you looking for...
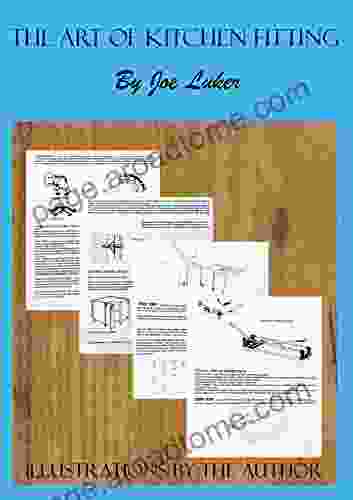

The Art of Kitchen Fitting: A Masterful Guide to Culinary...
The kitchen, the heart of...
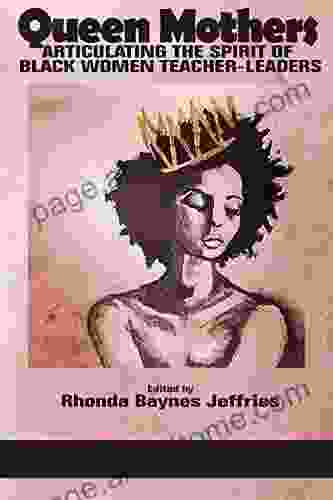

Articulating the Spirit of Black Women Teacher Leaders:...
In the tapestry of education,...
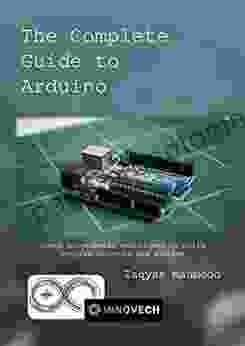

The Complete Guide to Arduino: Your Journey to...
: Unveiling the...
4.2 out of 5
Language | : | English |
File size | : | 11194 KB |
Text-to-Speech | : | Enabled |
Screen Reader | : | Supported |
Enhanced typesetting | : | Enabled |
Print length | : | 281 pages |